Note
Go to the end to download the full example code.
Simulating orientation selection effects in dipolar signals¶
An example on how to simulate dipolar signals with orientation selection effects.
Specifically, we simulate a dipolar signal with orientation selection effects arising from three different distributions of the orientation weights for a 4-pulse DEER dipolar signal simulated from a worm-like chain (WLC) distance distribution.
The orientation weights distributions are modelled as a 4-point spline with zero-derivative.
# Import the required libraries
import numpy as np
import matplotlib.pyplot as plt
import deerlab as dl
from scipy.interpolate import make_interp_spline
# Simulation parameters
reftime = 0 # Refocusing time, μs
conc = 50 # Spin concentration, μM
moddepth = 0.4 # Modulation depth
contour = 4.0 # Contour length, nm
persistence = 5.0 # Persistence length, nm
rmin,rmax = 1.5,6 # Range of the distance axis, nm
Δr = 0.050 # Distance resolution, nm
tmin,tmax = -0.5,5 # Range of the time trace, μs
Δt = 0.016 # Time resolution, μs
# Experimental time vector
t = np.arange(tmin,tmax,Δt)
# Distance vector
r = np.arange(rmin,rmax,Δr)
# Orientation selection distribution (must be a function defined in the range θ=[0,pi/2])
def Pθ_fcn(θ,Pθspline):
splineθ = [0, 0.4, 1, np.pi/2]
# Model P(θ) as a 4-point spline with zero-derivative at the edges
Pθ = make_interp_spline(splineθ, Pθspline, bc_type='clamped')
return 1-Pθ(θ)
# Spline parameters for the orientation selection distribution
spline_parameters = [
[0, 0, 0, 0], # Uniform, no orientation selection
[0.19, -0.12, 0.35, -0.163], # Least weighted θ=1
[0.19, -0.12, -0.25, 0.33] # Least weighted θ=0 and θ=pi/2
]
# Prepare the figure and axes
plt.figure(figsize=[8,4])
ax1 = plt.subplot(121)
ax2 = plt.subplot(122)
# Define custom colors for the plot
black = '#000000'
green = '#3cb4c6'
red = '#f84862'
colors = [black,red,green]
# Loop over the three cases
for spline_param,color in zip(spline_parameters,colors):
# Define the orientation selection distribution function
Porisel = lambda θ: Pθ_fcn(θ, spline_param)
# Construct the dipolar signal model
Vmodel = dl.dipolarmodel(t,r,Pmodel=dl.dd_wormchain,orisel=Porisel)
# Simulate the signal with orientation selection
Vsim = Vmodel(contour=contour, persistence=persistence, reftime=reftime, mod=moddepth, conc=conc, scale=1)
# Plot the simulated orientation selection distribution
θ = np.linspace(0,np.pi/2,300)
ax1.plot(θ,Porisel(θ),color=color,lw=2)
# Plot the simulated signal
ax2.plot(t,Vsim,color=color,lw=2)
# Format the figure
ax1.set_xlabel('Interspin vector orientation $θ$ (rad)')
ax1.set_ylabel('P(θ)')
ax2.set_xlabel('Time (μs)')
ax2.set_ylabel('V(t)')
plt.show()
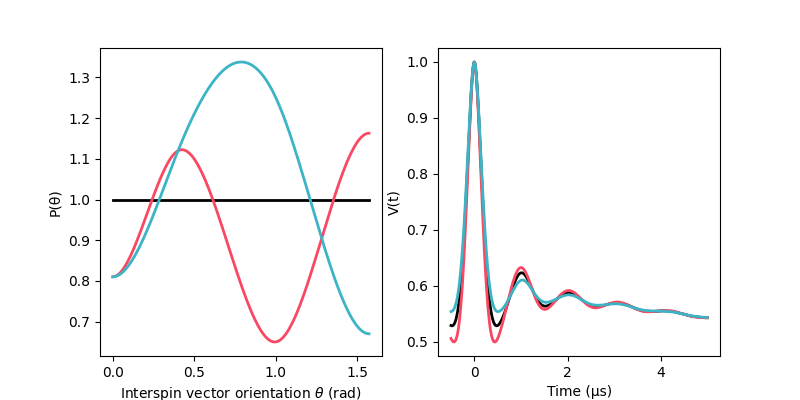
Total running time of the script: (0 minutes 1.323 seconds)